What Are Datasets And How Can They Benefit Your Business?
There was a time when only huge enterprises had the money and resources to leverage data for critical business decisions. Today, it’s possible for companies of any size to take advantage of data analytics with public and private datasets. Big data is no longer the exclusive realm of prominent market players.
Smaller companies often benefit most from datasets and big data. What is a dataset, and why are they so popular? They’re collections of information that are easier to work with, making it possible to execute data-driven business decisions more quickly. Thanks to more affordable technology, business leaders can use data to help them accomplish important business objectives, like improving customer service or making operations more efficient.
This guide will walk you through datasets and how to use them to your advantage. You’ll also learn how to prepare them for analysis and determine what data is relevant to your business practices.
What Are Datasets?
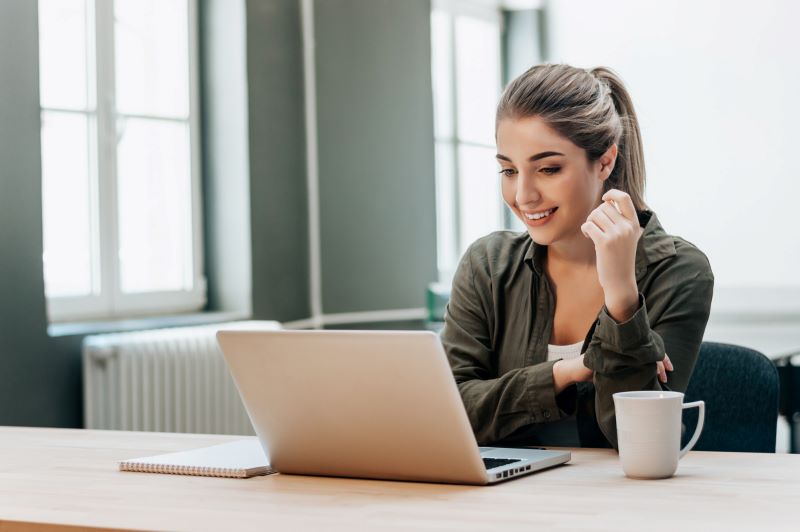
Datasets are organized collections of data typically associated with a specific topic. For example, a company might have one dataset containing the names of sales contacts and another that covers the sales figures for a particular period. They contain essential information needed by business analysts to come up with the kind of insights that help business leaders make decisions about the direction of their company.
Datasets can include the following types of information:
- Images
- Text
- Audio recordings
- Numerical values
One of the great things about datasets is that they can be used in almost any profession. Scientists use them to help analyze the environment or look at the findings from new biological samples. Retailers use the information collected on customers to determine ways of increasing sales by figuring out which products are most appealing. These days, it’s hard to find an industry that doesn’t rely heavily on datasets to improve performance in some way.
Are Datasets the Same as Databases?
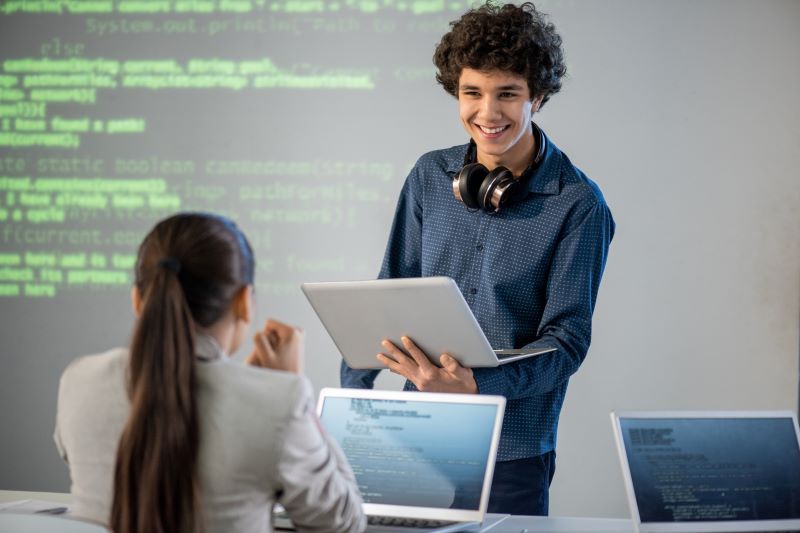
Datasets and databases are completely different entities. A dataset represents a specific collection of information around a particular area. They’re typically used to perform modeling and are smaller than a database. Applications for datasets include data visualization and statistical analysis, and many companies use datasets to train machine learning models and perform research.
Databases are a way of storing organized collections of data, including multiple datasets. Analysts can access the information by running a query using special tools and manipulating the data into a desired format.
Because databases are housed within a server, multiple users have access to the same information. Organizations typically store datasets in a database to make it easier to perform complex updates or other changes to the data. In addition, databases usually come with additional security and backup capabilities to protect information.
You can also store datasets in formats like a spreadsheet or CSV file. The goal is to organize the information to make it easier to analyze. You can create a dataset from various sources, including information from websites, by a web scraper supported by proxy servers. When you place datasets into an accessible format, sharing them, reproducing the data, or performing validation is easier.
Advantages Offered by Datasets
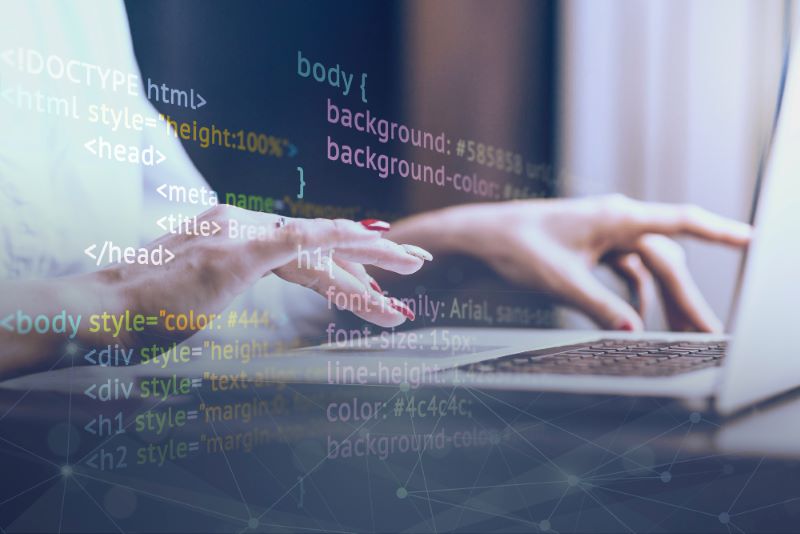
Why do so many businesses now look to big data to make themselves more efficient? Data analytics allow smaller companies to make decisions that help them compete with larger enterprises for market share. With the right insights, businesses can become more innovative and find ways to offer better prices and customer service.
Let’s look deeper at the benefits organizations can gain from dataset analysis.
Improved customer retention
While bringing in new business is great, you also want to do everything possible to keep the customers you have. Datasets help you observe the patterns of your customers and pick up on trends that might indicate they’re ready to move on to a competitor. You can get ahead of the curve by tailoring products to fit your customers’ needs and keep them loyal.
More targeted marketing
Instead of spending a lot of money on unfocused campaigns, smaller companies can refine their approach to go after specific customer segments. Datasets allow businesses to model their ideal customer and determine what kind of promotions they find most appealing.
More proactive risk identification
There’s an element of risk in every transaction. Datasets help you spot potential issues before they balloon into problems that harm your business and reputation. For example, if there’s a regular issue with the quality of a component you purchase from a vendor, you can cut them off and find another source before it impacts the products you create.
What Are the Different Types of Datasets?
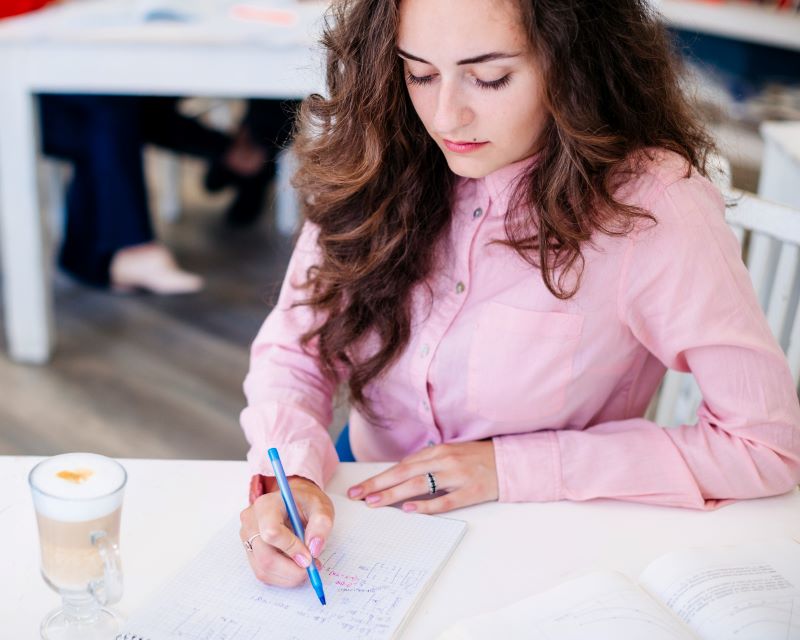
All datasets contain some value and a way to categorize the information. Below are examples of datasets often used for various purposes.
Categorical dataset
This dataset represents the different characteristics of a person or an object divided into groups. For example, an automobile company might use datasets to detail the features of a new car model they are rolling out or a potential buyer. Every categorical dataset comes with a qualitative value that takes in two values. For that reason, the qualitative value is often referred to as a dichotomous variable.
If there are more than two qualitative values, it is a polytomous variable. All values within a categorical dataset are assumed to be polytomous unless explicitly identified as dichotomous. An example of a qualitative value can include an individual’s gender (male, female, nonbinary) or marital status (married, unmarried, divorced).
Numerical dataset
Numerical datasets express information in numbers versus words or natural language. These datasets may also be referred to as quantitative datasets because they contain numbers. For example, companies might use numerical datasets to look at the specific performance of certain metrics expressed as a percentage, dollar amount, or other numerical value.
Examples of numeric data that you would find in this type of dataset include the following:
- The number of sales made during a specific quarter
- The page count of a newly released company white paper
- The number of new customers who signed up for a service from a specific region
Bivariate dataset
You typically see bivariate datasets used to model real-world situations. They contain only two variables and are often analyzed using scatterplots, simple linear regression, or correlation coefficients. Bivariate datasets can often be valuable in helping organizations determine how much profit they generate from different initiatives.
For example, businesses can use a bivariate dataset to model information on how much they brought in during consecutive sales quarters. One value would contain how much was spent on marketing during a specific period, while the other would include the total revenue generated during that same time. An analyst could then use a linear regression model to determine how much revenue the company raised for each marketing dollar spent.
Multivariate dataset
Multivariate datasets are used to hold at least three variables. For example, a real estate company could use one to store the features of a new model home being sold for a subdivision. It could contain values like the number of bedrooms, bathrooms, and floors.
Correlation dataset
Correlation datasets contain values demonstrating a specific relationship with each other. The correlation between two variables typically gets defined statistically. You might want to show a positive correlation, where both values go up or down in the same direction, or a negative one, where they go in opposite directions, depending on the circumstances. Sometimes a company may use a correlation dataset to show the lack of a relationship between two values or zero correlation.
You often see correlation datasets used by companies when they conduct market research. Figuring out which variables have the strongest relationships with each other, whether negatively or positively, helps companies make more informed decisions. Keep in mind that correlation datasets only show you that there is a relationship. Defining the type of relationship present requires additional regression analysis.
How Do You Create a Dataset?
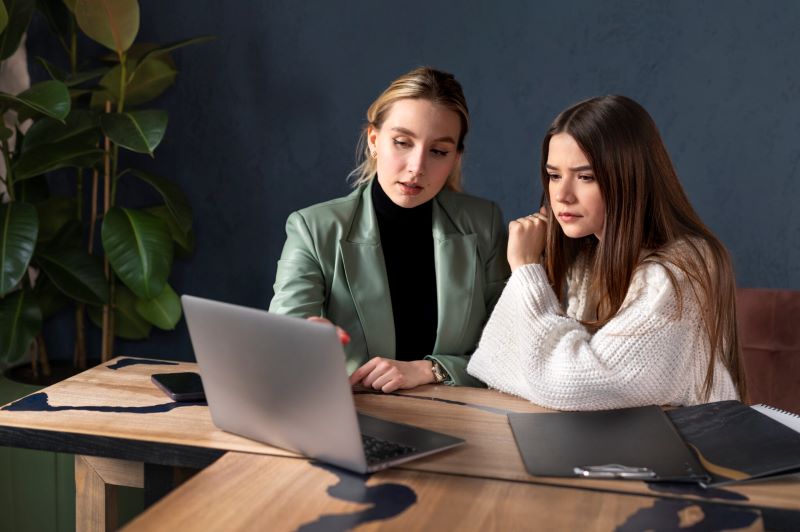
Many companies rely on web scraping technology to retrieve third-party data from external websites to use in datasets. Web scraping automatically collects unstructured information from sites, then stores the data in a structured format. For example, suppose an eCommerce retailer wanted to know how much a competitor was charging for similar products. In that case, they could send a web scraper to the site to collect pricing information to store in a dataset.
Your organization can connect with a third-party provider for data collection or invest in building a proprietary web scraping infrastructure. If you decide to do the latter, you’ll need the following tools.
Proxy servers
IP addresses get assigned to any device that accesses the internet. Proxy servers route requests from your web scrapers using their IP address versus the one assigned to your device. That way, the website sees only the proxy address, allowing your business to pull information anonymously.
Proxies let you crawl through websites more reliably and avoid getting banned. Using a web proxy also lets you send requests from specific regions to see content a website might display only for a given location. That’s essential for retailers who might want to gather product information from retailers in another part of the country.
When you set up a proxy pool or multiple proxies, you can send a higher volume of requests to cover your expanding dataset needs. In addition, proxy pools allow you to set up numerous concurrent sessions to access one or more websites. Another advantage offered by proxies is that you can avoid having a website owner issue a blanket IP ban.
Rayobytes offers a variety of proxies for companies to choose from. That way, you can ensure you have the firepower needed to keep your data solutions running as needed. From there, you can create datasets for further analysis.
Unlocking tools
As mentioned, some sites block web scraping tools for various reasons. It could be because they believe the automation consumes too many resources or want to avoid having their data taken by competitors. Companies typically use web unlocking tools and related algorithms to get around these roadblocks.
Unlocking tools help web scrapers achieve a higher success rate of capturing information from a targeted site. You can automate them to keep relaunching web scraping attempts. Some come with machine learning capabilities that adapt to the way websites initiate blocking.
Data collection software
You’ll need quality data collection software to retrieve and store your information electronically. Many data collection apps work on mobile devices like tablets or smartphones. You can build a tool or purchase one from a third-party vendor. Look for software with the capabilities to cover your specific business use cases.
You should also look at how well the tools work with other software already used within your company. Consider whether you want to pull information in batches or retrieve data in real time. Many low-code and no-code solutions can accommodate your company’s web scraping and data collection needs.
How Do You Prepare a Dataset?
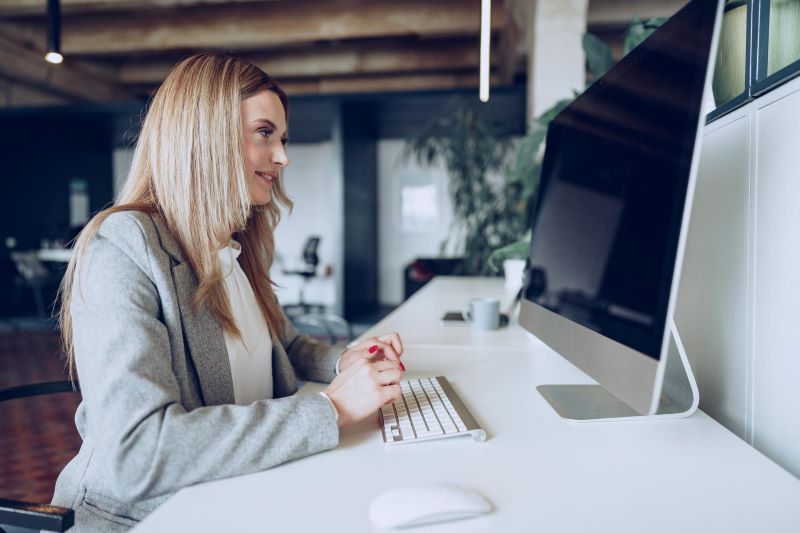
Once your business gathers various datasets, you’ll need processes to clean and manipulate the data into a usable format. First, you’ll want to put the information through multiple filters to ensure that it matches the specific characteristics you’re looking for. Next, create standardized data labels to make the cleaning process more manageable. There’s no reason to have values in your dataset that doesn’t represent what you’re looking for in your models.
From there, get rid of any duplicate entries. Start by figuring out how they made their way into the dataset. That will help you develop a more effective strategy for their removal. You may pull records from a database that get updated based on a specific identifier.
Therefore, you’d want to keep the most recent entry. However, that doesn’t necessarily mean you want to eliminate the older records. Instead, evaluate them to ensure there isn’t other valuable information you might want to transfer to your new dataset.
You’ll also want to account for any missing values in your rows and columns. If there are too many, it might be smarter to drop the entity. However, you’ll have to estimate the appropriate cutoff point where the information becomes unusable in your dataset.
Finally, make sure that your columns have the correct data type and values. If it’s a numerical column, that’s what you should have stored versus a text value. Fill in any missing values within your columns. Try to work through skewed data within a column by locating the median of a numeric value or the mode of a non-numeric one. That way, you don’t end up shifting the distribution of your information too far.
How Do You Keep Datasets Relevant?
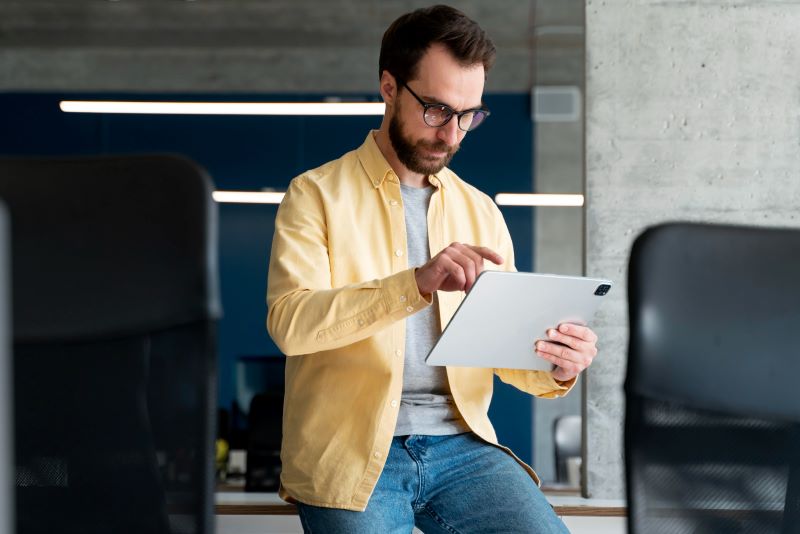
Generating independent datasets requires a significant investment. You’ll need to figure out which data points and records to locate, the sources for the information, and the technology needed for web scraping and other data collection efforts. In addition, your organization will need processes for validating data quality and ensuring that you aren’t missing any critical elements. You may also need to pull data from other sources, like an on-premises or cloud database, to enhance your data’s value.
One way to get around some of that is by leveraging existing datasets from the internet from an existing provider. Some companies still prefer to create an in-house infrastructure for collecting datasets. That means establishing a team for cleaning, structuring, and setting up pipelines to refresh datasets.
Regardless of whether you use preexisting datasets or set them up internally, you’ll need to keep the dataset relevant by:
- Updating dataset records periodically: Depending on your industry, you may need to refresh the information held in your datasets. For example, if you work in finance, you may want fresh stock market updates. Online vendors may wish to check the rankings of popular products daily, while marketers might want to determine the success of a marketing campaign a month to three months out.
- Figuring out what’s changed in a dataset: The information you collect can vary depending on the circumstances. An example is the average salary paid for a specific position. The average price of a product might drop depending on the market or environmental factors. If there’s been a significant shift in the values held within your datasets, that can influence how you carry out future business strategies.
- Keep up with historical data points: Make sure you’re tracking specific points that help you determine changes in consumer habits or repeating market cycles. Then, your analysts can use those markets to set up business models that provide you with the most current insights for making data-driven decisions.
What Techniques Can You Use To Learn More About Datasets?
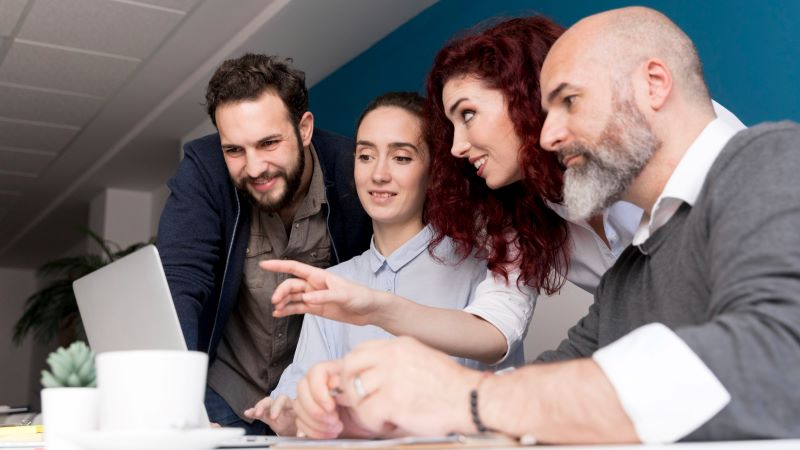
Once you have the information organized into a dataset, it’s easier for analysts to perform analysis and other mathematic operations that help leaders find value in the data held by their company. Below are examples of techniques typically used to gain more knowledge from datasets.
Mean
You use mean to determine the average of all observations from a dataset table. It works by setting up a calculation that divides the sum of all dataset observations by the total number of elements it holds. Mean equals the sum of all observations divided by the total of all dataset elements.
Median
The dataset median gives you the information in the middle of a dataset. You can find the median when you list a dataset in ascending or descending order. That value divides your dataset into two distinct halves.
Range
A dataset range will give you the difference between the lowest and highest value in the table. It’s used to measure how much the information varies using the same units used to measure the individual data values. The larger your range, the greater the variability in your dataset.
Unique value count
Unique values are those that only occur once within a dataset. Empty values are left out of the calculation.
Frequency count
The frequency count in a dataset calculates the total observations for every category contained in your dataset. For example, you can use frequency count to determine the percentage of customers who purchased a specific product after visiting a landing page versus those who navigated away.
Histogram
Analysts use histograms to divide potential values into classes or subgroups, giving them a visual representation of numerical data. Histograms consist of the following parts:
- Title: Describes the information within the graph.
- X-axis: Shows intervals representing the scale of values used for measurement.
- Y-axis: Shows how many times values happened within different intervals on the X-axis.
- Bars: The bar height within histograms tells how often values happen within intervals. In addition, the width of a histogram bar indicates the covered interval.
What Should You Look For in a Dataset?
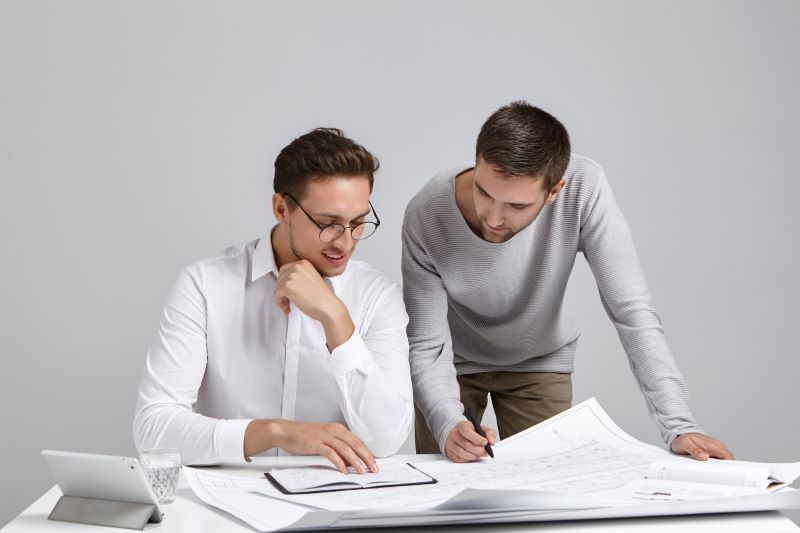
Before working with proprietary or externally obtained datasets, ask yourself the following questions to ensure you’re working with quality information.
Where did the information come from?
If you pulled the information from a web scraping tool, what website was used? How often is the information updated if the data comes from an internal database? Did you use the correct source of truth to fill up your dataset? Analysts may need additional formatting to ensure all attributes are consistent. Information in your datasets should be readable, comprehensive, and understood by those who use them as a resource.
How accurate is the data?
Look for elements that may stand out to see if they make sense in the context of the dataset’s other information. Analysts may have to make modifications to ensure that outliers don’t negatively affect their models.
Was the data cleaned?
Data often requires cleaning before it’s ready for analysis. That includes removing extra spaces, converting text to numbers, removing duplicate information, and dealing with empty values. A dataset containing too many empty values will be harder to model and analyze. In that instance, you may be better off discarding the dataset and setting up a new one with more reliable information.
Do you have enough data or too much data?
If you use your datasets for machine learning purposes, you’ll have to determine if you have too much or not enough information to meet your needs. For example, your project might require images, videos, or other data. If you don’t have enough information in your model, it may not give you the proper outcomes. Figuring out more complex problems typically requires a larger dataset.
Common Questions About Big Data
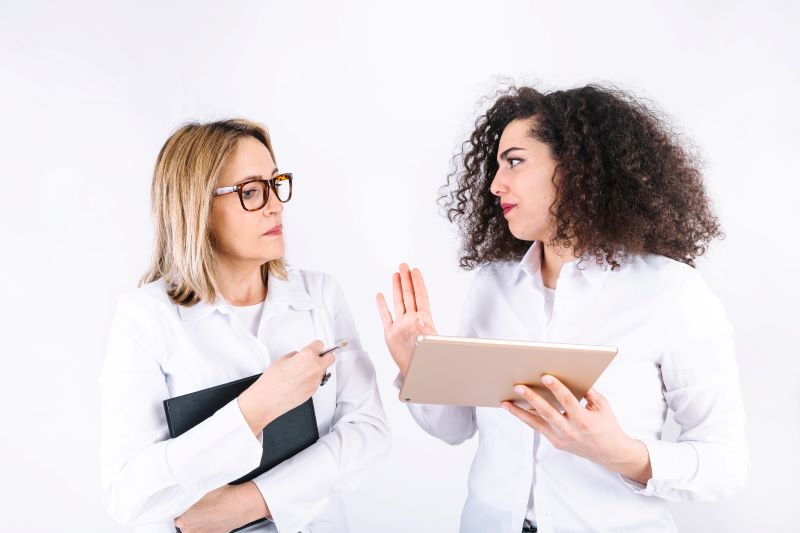
While much of the information covered in this article relates to the business use of datasets, below are some answers to broader questions about the subject.
What is a balanced dataset?
A balanced dataset contains roughly equal amounts of positive and negative samples. For example, if you had a dataset with two types of data, it would be balanced if there were around 50% of each. However, having only 20% of one item and 80% of another would be considered unbalanced. This isn’t always bad, depending on your intent for the dataset.
What is a dataset in machine learning?
Machine learning datasets contain information organized into three sets of data:
- The training dataset helps algorithms look for specific details in other datasets.
- The validation set contains good data that’s ready for use by an algorithm.
- The test set measures performance and adjusts as needed.
What is a training dataset?
A training dataset teaches algorithms how to find helpful information in another dataset.
What is a dataset in research?
Research datasets contain information collected from research projects, studies, images, and videos taken in the field. Researchers typically use datasets to validate survey results or perform follow-up analyses.
What is a dataset in statistics?
Statistical datasets contain values from observations made on samplings from specific populations. For example, a computer security company might create a dataset based on the results of a survey sent out to other companies about the state of their security infrastructure.
What is a dataset in Google Analytics?
Google Analytics users upload data using a dataset control and configure it as needed to support their analytical needs.
What is a feature dataset?
Feature datasets hold collections of related feature classes that share a standard system to coordinate values. For example, a transportation feature dataset might have related classes for roads, airports, and trains.
Final Thoughts
While figuring out what a dataset is and how to work with them may be daunting for companies unfamiliar with the process, the insights you gain can be invaluable to your business. In addition, setting up a data strategy for data collection, dataset preparation, and analysis can help leaders take their organization to the next level. Make sure you have what you need to support your data analysis processes by checking out Rayobyte’s selection of proxies.
The information contained within this article, including information posted by official staff, guest-submitted material, message board postings, or other third-party material is presented solely for the purposes of education and furtherance of the knowledge of the reader. All trademarks used in this publication are hereby acknowledged as the property of their respective owners.