Data Quality Metrics For Your Business
There’s no doubt that data is a critical part of any business. But all too often, businesses don’t have a good way to track the quality of their data. Without a way to track data quality, it can be difficult to identify and fix problems with your data.
It, therefore, means decisions are made based on low-quality data, which can lead to sub-optimal outcomes. The business might not be able to deliver on its promises, or worse, it could make decisions that adversely affect customers, employees, or other stakeholders. While it’s impossible to have perfect data, there are a number of ways to measure data quality and improve it in the long run.
That’s where data quality metrics come in. Data quality metrics allow you to track the quality of your data over time. This allows you to find and fix any problems before they become bigger issues. In this blog post, we’ll discuss examples of data quality metrics, how to measure data, and how to use them in your own business.
What Is Data Quality?
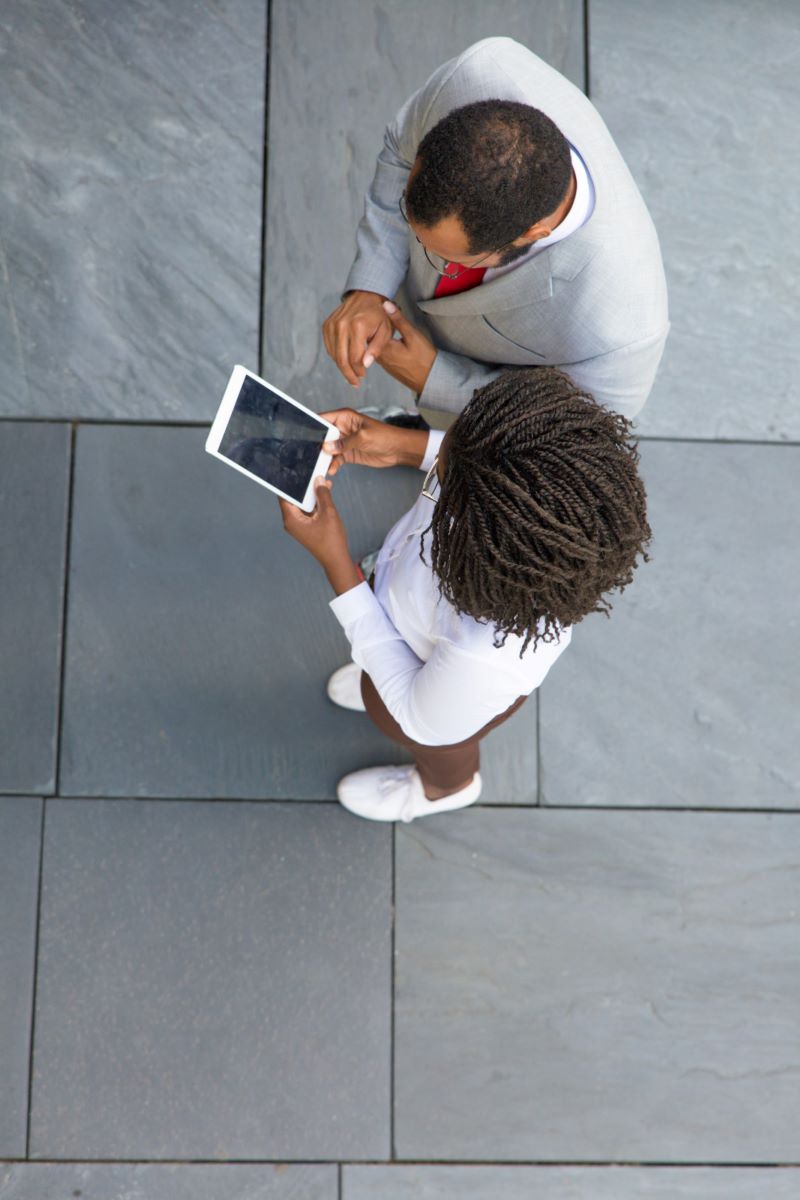
Data quality is the condition of a set of values of qualitative or quantitative variables. It is an assessment of whether the data is “fit for purpose” in a given context. Data quality attributes can be evaluated against explicit and implicit requirements to determine the fitness of the purpose.
It is important to note that data quality is not an inherent characteristic of the data itself, but rather a judgment made about the data in a particular context. In other words, we can have high quality data in one context and low-quality in another.
There are many factors that can impact the data quality attributes, such as the source of the data, the methods used to collect and store the data, the way the data is processed and analyzed, and the interpretative biases of the people using the data. Data quality assessment is a complex and iterative process that involves all these factors.
There are many ways to measure and assess data quality, but most methods can be broadly classified into two categories: objective and subjective measures.
Objective measures are based on well-defined criteria and can be quantitatively assessed. Subjective measures are based on the opinions of people and are generally more qualitative. Both objective and subjective measures have their strengths and weaknesses, and both are important to assess data quality.
What Is A Data Quality Metric?
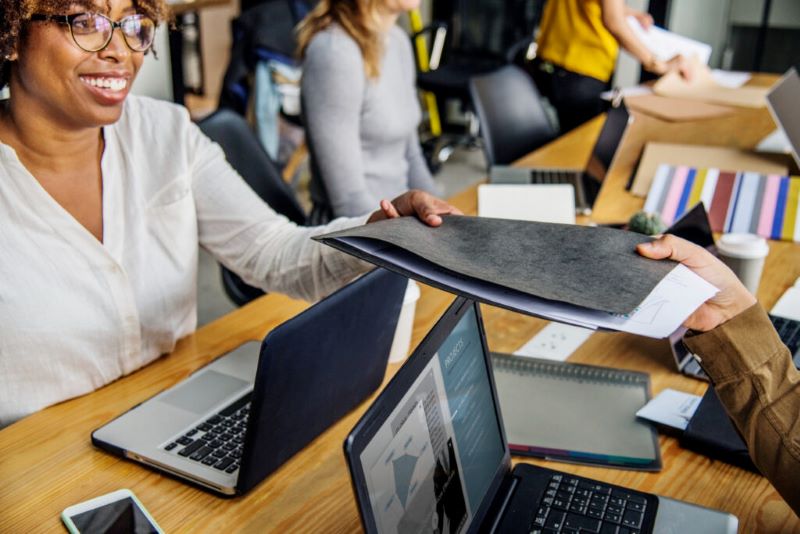
What is a data quality metric? A data quality metric is a measure of the accuracy and completeness of your data. It is important to have accurate and complete data to make sound decisions. There are many ways to measure data quality, but some common examples of quality metrics are discussed here.
Data Quality Metrics Examples
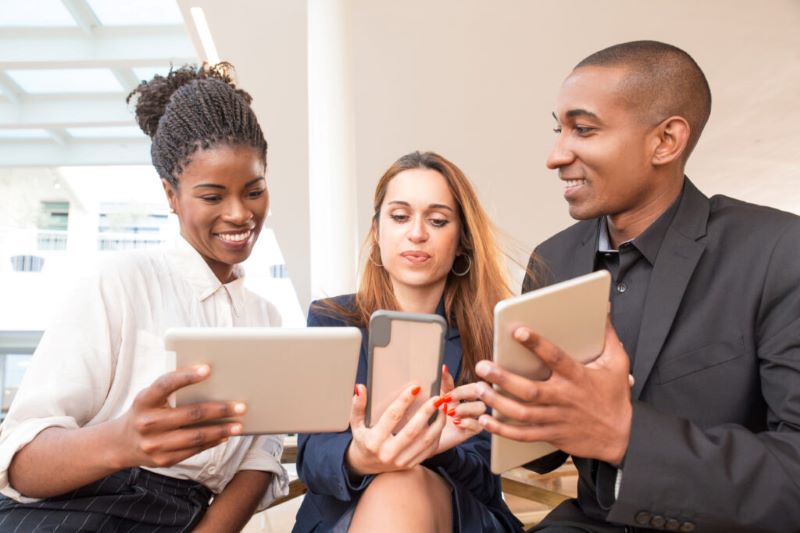
When discussing data quality metrics, it is useful to consider a set of metrics by which the quality of data can be evaluated. This can help provide a common language for discussing data quality issues and identify areas where improvement is needed. Data quality metrics can be measured in many ways. Here are some examples of metrics for data quality:
1. Number of errors
When it comes to data quality metrics, one of the most important metrics is the number of errors. This metric can help you determine how accurate your data is and how many problems need fixing. The number of errors can also be a good indicator of the quality of your data.
2. Number of warnings
Another important data quality metric is the number of warnings. This metric can help you determine how reliable your data is and how many potential problems there are. When there are many warnings, it can be an indicator that your data is not as reliable.
3. Number of invalid records
Invalid records are another important data quality metric. This metric can help determine how many records are invalid. Many factors can cause invalid records, such as incorrect, missing, or corrupt data.
4. Number of missing values
Missing values are another important data quality metric. This metric can help you determine how many values are missing and need filling in. Missing values occur when data is incomplete or missing altogether.
5. Number of duplicate values
Duplicate values are another important data quality metric. This metric can help you determine duplicated values that need removing. Duplicate values are due to errors in data entry or by data copied from one source to another.
6. Number of outliers
Outliers are another important data quality metric. This metric can help you determine how many values are outside the normal range and need investigating. Errors can cause outliers in data entry, when wrong data is entered. The data might also be legitimate, in which case it can give insights into unusual behaviors or events.
7. Range
When determining the quality of your data, the range is an important metric to consider. The range is the difference between the highest and lowest values in your data set. The range can help you determine the spread of your data and whether there are any outliers.
8. Average
The average is the sum of all values divided by the number of values. The average can help determine the central tendency of your data.
9. Median
The median is the middle value in your data set. Median is a good data quality metric to consider when there are outliers in your data set.
10. Error ratio
The error ratio is the number of errors divided by the total number of values. The error ratio as a data quality metric can help you determine the accuracy of your data.
Data Quality Dimensions
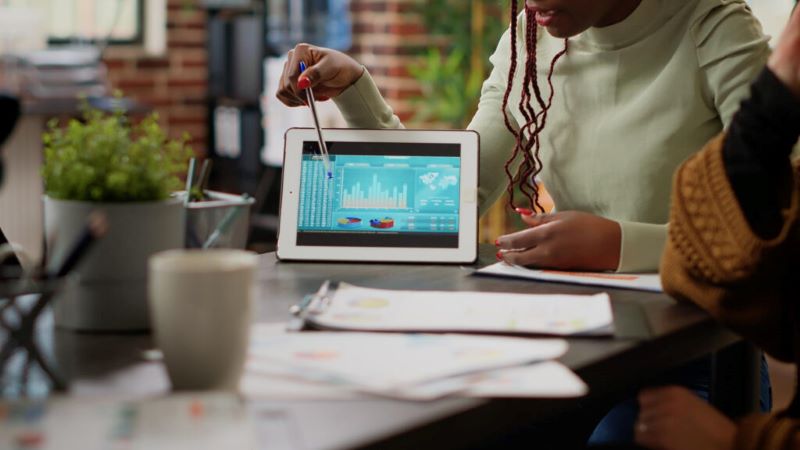
When discussing data quality attributes, it is important to consider the different dimensions. Quality dimension is a perspective or aspect from which data can be viewed. It is important to consider all these dimensions when seeking to assess data quality, as each can have different effects on how the data can be used.
Using data quality dimensions can help identify issues and prioritize improvement efforts. Data quality dimensions can also be used to create metrics, which are numerical measures that assess the quality of a given dimension.
The most common data quality dimensions are mentioned below.
Accuracy
Accuracy is a data quality measure of how well data represents the real world. Data can be accurate, but still not representative of the real world (for example, a sample of data from a small region may be accurate, but not representative of the larger population). Data can also be inaccurate, but still be representative (for example, a measurement that is imprecise but unbiased).
Data quality is often expressed in terms of accuracy and precision. Accuracy is a measure of how close data is to the real world, while precision is a data quality measure of how close data is to other data. For example, the use of different date formats (DD/MM/YYYY vs. MM/DD/YYYY) can lead to inaccuracy when data is exchanged between countries.
When data is inaccurate, it can lead to incorrect conclusions. For example, if data on the number of people in a city is inaccurate, this may lead to incorrect estimates of the city’s population. This, in turn, could lead to incorrect decisions about infrastructure and public services.
There are many ways to measure accuracy, but some common methods include:
- Measuring the agreement between two data sets: This can be done using techniques like inter-rater reliability, where two or more people rate the same data set, and the agreement between their ratings is calculated.
- Comparing data to a known gold standard: This is often done in medicine, where a new test is compared to the gold standard of a biopsy.
- Using statistical methods: Normal distribution can give you a sense of how accurate data is by measuring how close data is to the expected value.
Accuracy is important, but it is not the only thing that matters. Data can be accurate, but still not representative of the real world (for example, a sample of data from a small region may be accurate, but not representative of the larger population). Data can be inaccurate, but representative. For example, an entry in a database missing a decimal point is inaccurate, but the value may be close enough to the true value to be representative.
Completeness
Completeness is one of the data quality attributes that describes the extent to which data is complete. Data is considered complete if it contains all the required data elements, is free of errors and omissions, and is timely. Data completeness is important because it ensures that decision-makers have all the information they need to make informed decisions.
Data completeness is often measured as a percentage of required data elements present. For example, if a data set contains 100 required data elements, and 90 of those elements are present, the data set is considered 90% complete.
Data sets can be incomplete for various reasons, including errors and omissions in data collection and data entry, late or missing data, and incorrect or missing metadata. Data completeness can also be affected by the choice of data sources. For example, an entry in a database may be missing if the data source does not include the relevant information. In addition, data collected from different sources may be incompatible, making it difficult to combine the data into a single coherent dataset.
Data completeness is an important consideration in data quality assessment and data quality improvement efforts. Data quality assessments can help identify incomplete data sets and identify the causes of incompleteness. Data quality improvement efforts can then focus on addressing the causes of incompleteness.
There are various techniques that can be used to assess data quality completeness, including manual review, automated checking, and statistical analysis. Data quality assessment and data quality improvement efforts should be tailored to the specific needs of the organization and the data set in question.
Data completeness is a key dimension of data quality. Ensuring that data is complete is essential to ensure that decision-makers have all the information they need to make informed decisions. Data quality assessment and data quality improvement efforts should be tailored to the specific needs of the organization and the data set in question.
Consistency
As a dimension of data quality, consistency refers to the degree to which data values conform to an established standard. That standard can be set internally within an organization or externally by industry, regulatory body, or other entity. Consistency is important because it helps ensure that data can be accurately interpreted and used for decision-making.
There are several types of consistency that can be measured, including:
- Format consistency: Data values are formatted in the same way across different data sets.
- Structural consistency: Data values are organized in the same way across different data sets.
- Logical consistency: Data values conform to logical rules or relationships.
- Referential consistency: Data values can be cross-referenced against other data sets.
Consistency is often measured using some type of data quality metric, which can be either a quantitative or qualitative measure. A few common consistency metrics for data quality include:
- Percentage of missing values: This metric measures the percentage of missing data values from a data set. A high percentage of missing values can indicate that the data is inconsistent.
- Number of unique values: This metric measures the number of unique values (outliers) in a data set. Many unique values can indicate that the data is inconsistent.
- Number of errors: This metric measures the number of errors in a data set. Many errors can indicate that the data is inconsistent.
A high degree of consistency across different data sets can help increase the credibility of the data and the organization that collected it.
Timeliness
Data is timely if it is available when needed. For example, data about yesterday’s sales can be used today to make decisions about today’s sales. Data that is not timely is of little use.
There are two aspects to timeliness: currency and latency. Currency refers to how up-to-date the data is. Latency refers to how long it takes for the data to become available.
Currency
Currency is the dimension of data quality that refers to the timely nature of information. For data to be considered current, it must be accurate as of the time it is accessed or used. Currency is important because outdated information can lead to incorrect decisions.
There are two types of currency: static and dynamic. Static data is not expected to change over time, such as a product catalog. Static data can become outdated, but it does not need to be updated regularly. Dynamic data, on the other hand, is expected to change frequently, such as stock prices. Dynamic data must be regularly updated to be considered current.
Currency is important for both individuals and businesses. For individuals, currency is important for making personal decisions, such as whether to buy or sell a stock. For businesses, currency is critical for strategic decisions, such as where to allocate resources.
There are many ways to ensure that data is current. Data can be manually updated, or automatically updated using a process known as data refreshing. Data refreshing is the process of automatically updating data regularly. Data can also be updated in real-time, which is the process of updating data as it changes.
Ensuring that data is current is important for making accurate decisions. There are a number of ways to ensure that data is current, including manual updates, data refreshing, and real-time updates. By keeping data current, businesses and individuals can make informed decisions that lead to better outcomes.
To ensure data currency, organizations should establish policies and procedures for regularly refreshing information. For dynamic data, real-time updates may be necessary. Data currency can be monitored through auditing and feedback mechanisms. By keeping data current, organizations can improve the quality of their decision-making.
Auditability
Auditability is a key dimension of data quality. Data that can be tracked and traced back to its source is said to be “auditable.” This traceability is important to ensure the accuracy and completeness of data. Auditable data can be used to reconstruct past events and understand how they unfolded. This is valuable for compliance purposes, root cause analysis, and problem solving.
Auditability is also an important consideration in data governance. Data that is not auditable is more likely to be misused or misunderstood. There are many ways to make data more auditable. One common approach is to add “metadata,” or information about the data, to help explain its origins and context.
Another approach is to create logs of all changes to the data. This can be done automatically, through software that records every change made. Making data auditable can be costly and time-consuming. However, the benefits of auditable data usually outweigh the costs. Auditable data is more likely to be accurate and complete and can help organizations avoid problems down the road.
Uniqueness
Uniqueness is a data quality measure that assesses the degree to which each record in a dataset is distinct from every other record. A dataset is said to have high uniqueness if each record has a high degree of individuality, that is if no two records are exactly alike. A dataset is said to have low uniqueness if there are many duplicate records or if the records are very similar.
There are many ways to assess data quality uniqueness, but one common method is to calculate the percentage of duplicate records in a dataset. This can be done by comparing each record to every other record in the dataset and counting the number of times a match is found. The percent of duplicate records is simply the number of matches divided by the number of records in the dataset.
Another way to measure uniqueness is to calculate the entropy of the dataset. Entropy is a data quality measure of the variability of a dataset and is often used in statistics and data mining. A dataset with high entropy has many distinct values, while a dataset with low entropy has few distinct values.
Uniqueness is important because it is often used as a measure for data quality. Data that is not unique is often less valuable, as it may be difficult to distinguish one record from another. Data that is highly unique is often more valuable, as it is easier to identify individual records.
Uniqueness is also important because it can impact the accuracy of data analyses. Data that is not unique is more likely to contain errors, as it is more difficult to determine which values are correct. Data that is highly unique is less likely to contain errors, as it is easier to identify the correct values.
Uniqueness (homogeneity and heterogeneity of data) is an important dimension of data quality and should be considered when assessing the quality of a dataset. Datasets with high uniqueness are often more valuable and accurate than those with low uniqueness.
Validity
The dimension of validity determines whether the data meets the requirements for its intended use. There are three types of validity: content, face, and construct. Content validity is a judgment of how well the data represents all the important aspects of the phenomenon being measured. Face validity is a judgment of how well the data appears to measure what it is supposed to measure. Construct validity is a judgment of how well the data measures the theoretical construct it is supposed to measure.
There are several ways to establish validity, including:
- Review by experts: Experts in the field can review the data to determine if it is valid for its intended use.
- Statistical methods: Statistical methods can be used to assess the validity of the data.
- Comparison to other data: The data can be compared to other data sets to assess its validity.
The dimension of validity is important because it determines how accurately the data represents the phenomenon being measured. If the data is not valid, it can lead to inaccurate conclusions.
Conformity
Conformity is one of the data quality attributes that refer to the degree to which data adheres to certain standards. In other words, it measures how well data fits within pre-established norms. There are many types of standards that data can be compared against, including but not limited to:
- Industry standards
- Organizational standards
- Technical standards
Conformity is important because it helps ensure that data is consistent and comparable. When data is not in compliance with standards, it can create problems such as inconsistency, incompatibility, and errors.
There are a few ways to measure conformity. One common method is to use a conformity index, which assigns a numerical score to data based on how well it adheres to standards. Another option is to use a compliance checker, which is a tool that automatically checks data against standards and identifies non-conforming values.
There are many ways to improve conformity. One option is to establish clear standards that data must meet. Another option is to use data cleansing and validation techniques to ensure that data meets standards. Finally, it is also possible to use data transformation techniques to convert non-conforming data into a compliant format.
Importance of Data Quality Dimensions
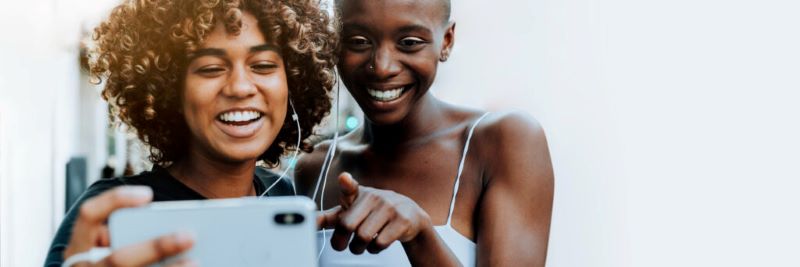
Information is critical to business success. The value of information decreases as its accuracy and timeliness decrease. Data quality dimensions are characteristics of data that describe its fitness for use. They help organizations understand the value of their data and take steps to improve it. Once data quality dimensions are understood, it is easier to identify problems and correct them. This, in turn, leads to improved business decisions, reduced costs, and increased revenues.
They are important due to the following reasons:
- Reduced the cost of bad data: Data quality problems can cost organizations a lot of money. Inaccurate data can lead to lost opportunities, wasted resources, and even legal penalties. By understanding and improving metrics for data quality, organizations can reduce these costs.
- Improved decision making: High quality data is essential for making sound business decisions. Poor data can lead to bad decisions that can cost organizations dearly. By understanding and improving data quality, organizations can make better decisions.
- Increased revenue: High quality data can help organizations sell more products and services and charge higher prices. Poor data can lead to lost customers and lower sales. By understanding and improving data quality, organizations can increase their revenues.
- Improved customer satisfaction: High quality data can help organizations provide better customer service. Poor data can lead to unhappy customers and lower satisfaction levels. By understanding and improving data quality, organizations can improve customer satisfaction.
Data quality is important for all organizations, regardless of size or industry. By understanding and improving data quality, organizations can improve their bottom line.
Measuring Data Quality
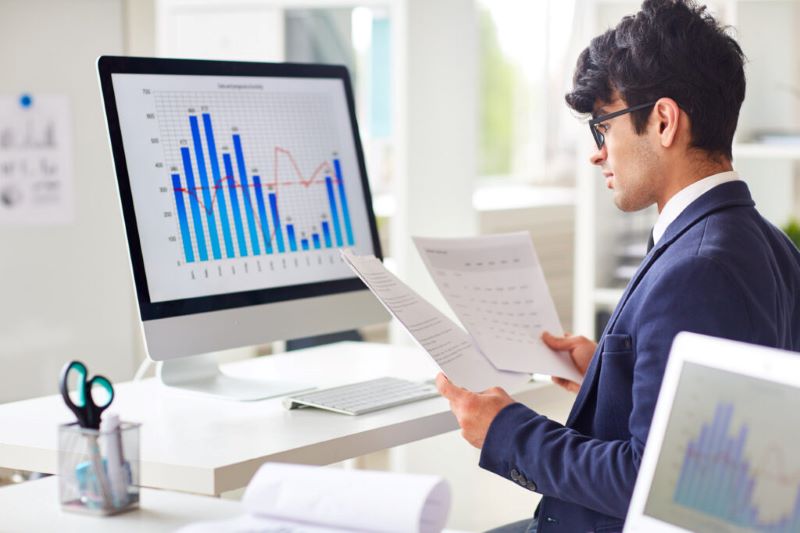
Data quality is the process of measuring how accurate, consistent, and complete your data is. This can be done through manual processes or automated software. Data quality is important because it helps organizations make better decisions, improve efficiency, and prevent errors. There are many factors that impact data quality, including data entry, data cleansing, and data validation.
Organizations can measure data quality in many ways, including manually or through automated software. Data quality is important because it helps organizations make better decisions, improve efficiency, and prevent errors. When measuring data quality, organizations should consider factors such as data entry, data cleansing, and data validation. By taking the following steps, organizations can improve the quality of their data and make better decisions.
Define the standard of quality data
When discussing quality data, it is important to establish a baseline or standard of what qualifies as quality data. This allows organizations to measure their data against a set criterion and work to improve their data quality as needed.
There are a few ways to establish a standard for quality data. One is to develop organization-specific standards that data must meet to be considered high quality. Once these standards are established, data can be measured against them to see if it meets the criteria.
Another way to establish a standard for quality data is to use industry-wide standards. These are standards established by professional organizations or groups to provide a benchmark for quality data. These standards can be used to measure the data of any organization, regardless of size or industry.
Once a standard for quality data has been established, it can be used as data quality measure of any organization and to improve their data as needed. By having a standard, organizations can ensure that their data is of the highest quality possible.
Determine how often data should be verified for accuracy
There are a few factors to consider when determining how often data should be verified for accuracy. The first is the importance of the data. If the data is critical to decision-making, it should be verified more frequently. The second factor is the stability of the data. If the data is subject to change, it should be verified more often. The third factor is the cost of verifying the data. If the cost of verifying the data is high, it should be verified less frequently.
In general, data should be verified at least once a month. More frequent verification may be necessary for critical data or data subject to change. For other data, less frequent verification may be sufficient.
Set up processes to ensure data is entered correctly the first time
Incorrect data entry is a common problem that can lead to inaccurate information stored in a database. This can then cause problems when trying to use that data for decision-making or other purposes. To avoid this, it is important to implement processes that ensure that data is entered correctly the first time.
One way to do this is to use data entry forms that include built-in validation. This means the form will check that the data being entered meets the necessary requirements before it is accepted. For example, a form may check that a date is in the correct format or that a number is within a certain range.
Another way to ensure data quality is to use drop-down menus for fields with limited options. This helps ensure that the data entered is correct, as it is impossible to type in a value that’s not in the list.
It is also possible to use data entry rules to check that the data entered meets certain criteria. For example, a rule could check that a date is in the future or that a postcode is in the correct format. Data quality is important for all businesses. So, it is worth taking the time to implement processes that ensure accurate data entry. By doing so, you can avoid the problems caused by incorrect data.
Use data profiling to identify data quality issues
Data profiling is the process of examining a dataset to identify patterns and trends. It can be used to identify data quality issues, such as missing values, incorrect values, and outliers.
Data profiling can be used to measure data quality in many ways. For example, you can use it to calculate the percentage of missing values in a dataset. You can also use it to identify incorrect values, such as values that are too high or too low.
Outliers can also be identified using data profiling. An outlier is a value significantly different from the rest of the values in a dataset. Outliers can be caused by errors in data entry, or they can be legitimate values that are rare.
Data profiling is a valuable tool for measuring data quality. It can help you identify issues that need to be fixed, and it can also help you understand the data better.
Regularly review and update data cleansing processes
As a way to measure data quality, regularly review and update your data cleansing processes. This helps ensure your data is as clean and accurate as possible, and that you can identify and fix any issues that may arise.
There are a few ways to go about this. First, you can regularly review your data cleansing process, making sure to update and improve it as needed. This may involve adding new steps, changing existing ones, or even removing steps no longer necessary.
Another approach is to periodically audit your data cleansing process. This can be done internally, by someone within your organization, or externally, by an independent third party. An audit can help you identify areas where your process could be improved and can give you a chance to make changes before problems arise.
Finally, you can also use data quality metrics to measure the effectiveness of your data cleansing process. By tracking these metrics for data quality, you can identify areas where your process is falling short and make changes to improve it.
Why Measure Data Quality?
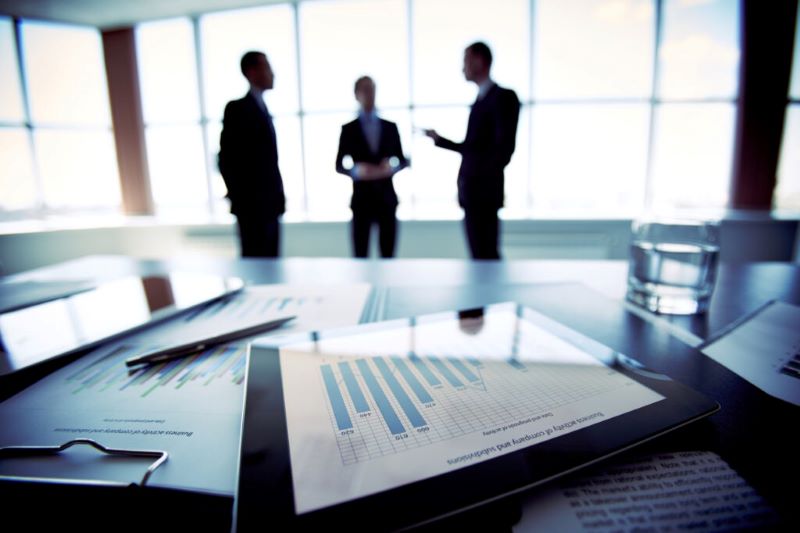
Data collection is an expensive and time-consuming process. So, it is important to ensure collection of high quality data. Once quality data has been collected, it is essential to measure it to ensure the dataset is useful. Data quality metrics help identify areas where improvement is needed and can also be used to track progress over time.
Measuring data quality is important for many reasons.
First, data quality directly impacts business performance. Poor data quality can lead to bad decision-making, which can lead to decreased revenue, decreased market share, and even bankruptcy.
Second, data quality is a measure of the quality of big data initiatives. If the data collected is poor, then the insights gleaned from that data will also be of poor quality. Organizations must ensure that they have high quality data if they want to realize the benefits of big data.
Third, measuring data quality can help organizations identify and fix problems early on. The sooner a problem is identified, the less costly it will be to fix. When data quality issues are left unaddressed, they can snowball into larger and more expensive problems.
Fourth, measuring data quality is essential in meeting regulatory requirements. For example, the General Data Protection Regulation requires organizations to take measures to ensure the accuracy of personal data. It is the responsibility of organizations to ensure they measure data quality and take steps to improve it.
Finally, measuring data quality can help organizations benchmark their performance. By tracking data quality metrics, organizations can see how they compare to their peers and identify areas where they need to improve.
Measure and Track The Quality Of This Data
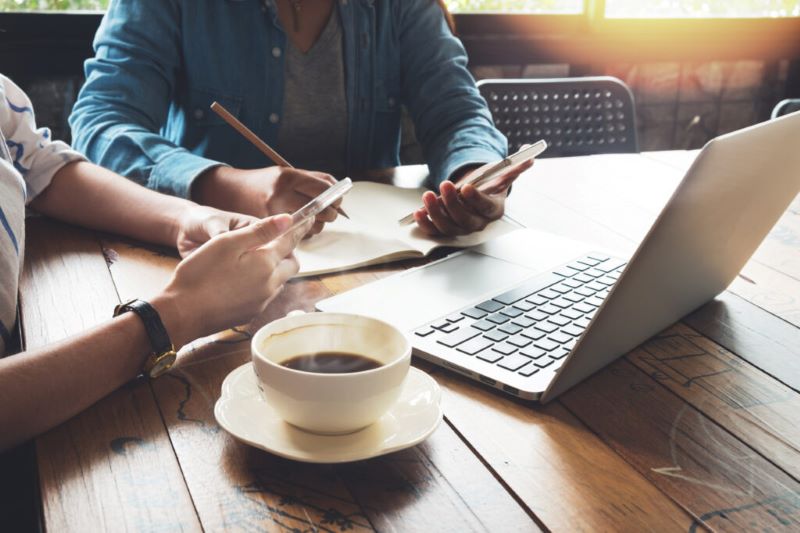
Data quality can be measured in many ways. One common method is to track the number of errors that occur during data entry or data processing. This helps identify areas where data quality needs to be improved.
Another way to measure data quality is to regularly review and update data cleansing processes. This helps ensure that data is as clean and accurate as possible and that any issues can be identified and fixed before they cause problems.
Finally, data quality metrics can be used to track the effectiveness of data cleansing processes. By tracking these data quality metrics, you can identify areas where your process is falling short and make changes to improve it.
Measuring data quality is important for all businesses, as it can help identify areas where improvements need to be made. By taking the time to measure and track data quality metrics, you can avoid the problems that can be caused by incorrect or dirty data.
Web Scraping Data Using Proxies
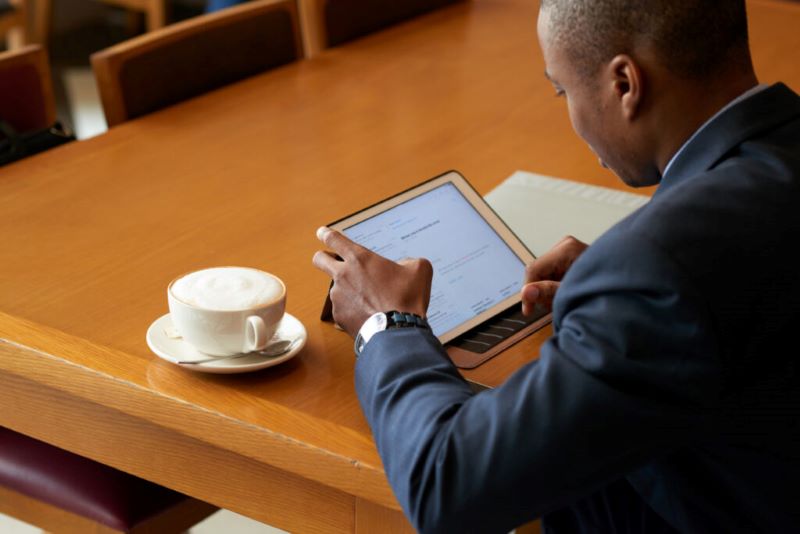
Data is essential for any business or individual seeking to make informed decisions. Web scraping is one of the most effective ways to collect data. It is defined as the process of extracting data from websites. Web scraping can be used to collect pricing data, product data, contact information, reviews, and much more.
Proxies are a crucial asset to web scraping, as they enable high-performance data collection. Proxies can be divided into two main categories: data center proxies and residential proxies. Data center proxies are further divided into dedicated, semi-dedicated, and rotating proxies. Residential proxies are further divided into static and rotating proxies.
Final Thoughts
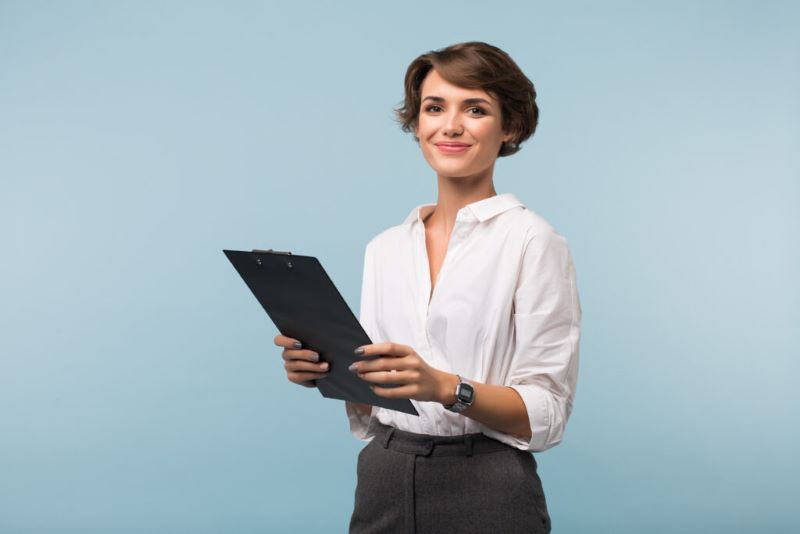
When looking for the best proxies for web scraping, it is important to consider the type of data you are trying to collect, the performance you need, and your budget. Data quality is an important metric to consider, as is the reliability of the proxies you use. Data quality metrics are essential in ensuring that the data you collect is accurate and can be used to improve decision making.
Rayobyte is a proxy provider that offers both data center and residential proxies. Their residential proxies are some of the most competitive in the market, with plans starting at 15 GB per month at $15. Their data center offerings are perfect for those who need many IP addresses and are willing to pay a premium for them. Plans start at $247.5 per month for 99 IP addresses.
No matter what your needs are, Rayobyte has a proxy solution that fits your budget and requirements. So, if you’re looking to scrape data effectively, consider Rayobyte a great option for high-quality reliable proxies.
The information contained within this article, including information posted by official staff, guest-submitted material, message board postings, or other third-party material is presented solely for the purposes of education and furtherance of the knowledge of the reader. All trademarks used in this publication are hereby acknowledged as the property of their respective owners.