Big Data VS. AI: Understanding The Key Differences
In today’s data-driven world, two terms constantly grab headlines: Big data and artificial intelligence (AI). Often used interchangeably, these technologies are revolutionizing every aspect of our lives. But what exactly are they, and what are the key differences between big data vs. AI?
This article delves into the mysteries of big data and AI, exploring their unique strengths and how they work together as a powerful force for innovation. Below are the most important distinctions and similarities.
What Is Big Data?
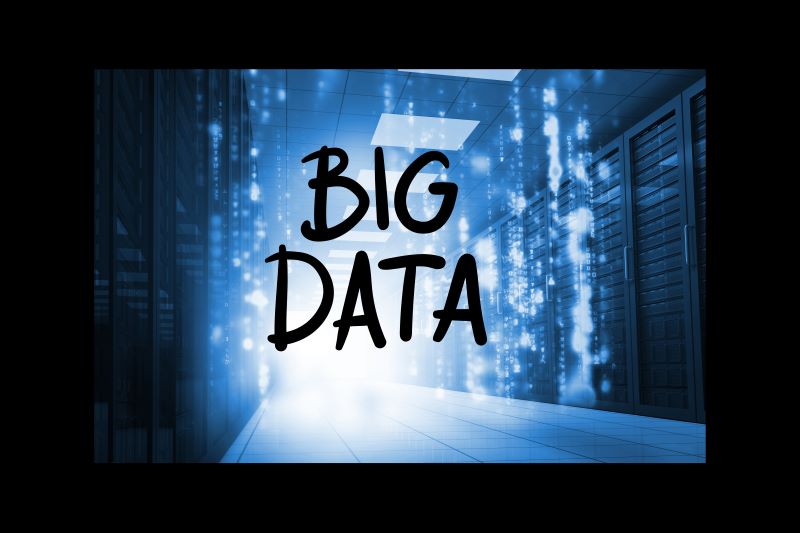
The term “big data” refers to massive and complex datasets that traditional data processing tools often struggle to handle. It’s not just about the amount of data but also the variety and speed at which it’s collected.
Big data is typically characterized by the 5 Vs — Volume, variety, velocity, veracity, and value:
- Volume: The amount of data is enormous. Think massive social media feeds, sensor data from millions of devices, or financial transactions happening every second.
- Variety: The data comes in all sorts of formats, from structured data in tables to unstructured text, images, and videos.
- Velocity: The data is constantly growing and changing at an ever-increasing rate.
- Veracity: Refers to the quality and reliability of the data. Big data often includes noisy, incomplete, or inconsistent data, making it crucial to ensure data quality to derive accurate insights.
- Value: The ultimate goal of big data analysis is to derive actionable insights and value from the data. Extracting meaningful insights from large datasets can lead to improvements in decision-making, innovation, efficiency, and competitiveness for businesses and organizations.
Big data can be sourced from various channels and platforms across both digital and physical environments. Common sources of big data include the following:
- Social media: Popular social media platforms generate extensive amounts of data in the form of posts, comments, likes, shares, and much more. This data can provide insights into user behavior, preferences, sentiments, and trends.
- Internet of Things (IoT) devices: IoT devices include sensors, wearables, smart appliances, industrial equipment, and vehicles that generate real-time data streams. This data includes information about environmental conditions, device performance, user behavior, and more.
- Online transactions: e-commerce platforms, banking systems, and financial institutions generate large volumes of transactional data. This data includes purchase history, payment details, user demographics, and other transaction-related information.
- Web logs and clickstream data: Websites and online platforms generate log files and clickstream data that record user interactions, website navigation paths, clicks, page views, session durations, and other browsing behaviors. Analyzing this data can provide insights into user engagement and website performance.
- Mobile devices: Mobile apps and devices generate data such as location information, app usage patterns, device activity, preferences, and user interactions. Mobile data can provide valuable insights for personalized marketing, location-based services, and user behavior analysis.
- Machine and sensor data: Industrial equipment, manufacturing systems, and infrastructure are equipped with sensors that collect data on temperature, pressure, vibration, energy consumption, and other parameters. Analyzing this data enables predictive maintenance, process optimization, and quality control.
- Multimedia content: Images, videos, and audio files contribute to big data through social media platforms, multimedia websites, surveillance systems, and digital entertainment services. Analyzing multimedia content requires specialized techniques such as image recognition, video analysis, and speech processing.
- Public data sources: Government agencies, research organizations, and public institutions provide access to various datasets, including demographic data, economic indicators, healthcare statistics, weather data, and geographic information. These public datasets are valuable for research, analysis, and decision-making.
- Customer interactions: Customer service interactions, call center logs, emails, chat transcripts, and customer feedback provide valuable insights into customer satisfaction, support issues, product feedback, and market trends.
- Research and scientific data: Scientific experiments, research studies, genomic data, astronomical observations, and simulations generate large volumes of data. Analyzing research data can lead to discoveries, insights, and advancements in various fields.
What Is AI?
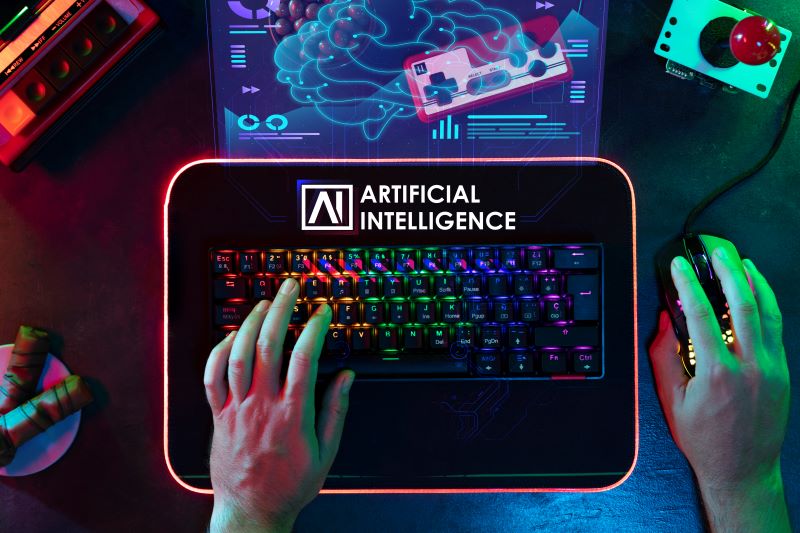
Artificial intelligence is an area of computer science focused on creating intelligent machines that can perform tasks that typically require human intelligence. Examples of these tasks include the following:
- Interpreting speech: An example could be the virtual assistants on your phone that understand your voice commands.
- Playing games: Think chess-playing computers or AI bots that can beat humans at complex games like Starcraft II.
- Identifying patterns: This is a core function of many AI applications, from spam filters that recognize unwanted emails to recommendation systems that suggest products you might be interested in.
AI systems typically learn by processing large amounts of data and identifying patterns within it.
There are different approaches to AI, but some of the most common include machine learning, which involves training algorithms on data so they can learn to perform specific tasks without being explicitly programmed, and deep learning, a type of machine learning that uses artificial neural networks, which are inspired by the human brain’s structure, to process information and make decisions.
Generative AI overview for project managers
Generative AI is a branch of AI focused on creating entirely new content. This content can come in many forms, including the following:
- Text: Think of writing assistants that can craft realistic dialogue, poems, or even scripts based on your instructions.
- Images: Generative AI can create new photos and art styles or even alter existing images to your specifications.
- Audio: Composing music in different genres or generating sound effects are both within the realm of generative AI.
Many AI project management tools use generative AI to write, summarize data, create emails, etc.
Big Data vs. AI: What’s the Difference?
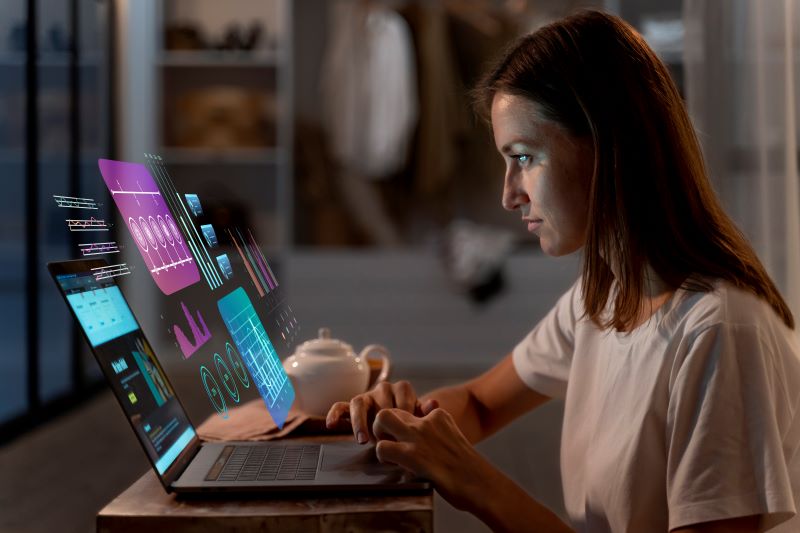
Big data and AI are two powerful tools that can work together, but they serve different purposes.
Think of big data as a giant warehouse full of information. It’s a massive collection of data in all shapes and sizes, from structured numbers to messy text and videos.
Big data itself doesn’t analyze anything. It’s just the raw material waiting to be processed.
Now, imagine AI as the factory that takes the big data and turns it into something useful. AI uses algorithms to analyze patterns, make predictions, and even learn and improve on its own.
AI needs big data to function. The more data it has, the better it can learn and perform its tasks.
Still confused? Here’s a more detailed breakdown of the differences between AI vs. big data:
Focus
Big Data focuses on the collection, storage, management, and analysis of large volumes of data to extract insights, patterns, and trends.
AI, on the other hand, focuses on developing systems or algorithms that can perform tasks that typically require human intelligence, such as understanding natural language, recognizing patterns in data, making predictions, and autonomous decision-making.
Purpose
Big data primarily aims to uncover insights, correlations, and trends hidden within vast datasets to inform decision-making, optimize processes, improve efficiency, and gain a competitive advantage.
Conversely, AI aims to develop systems or algorithms that can perform tasks autonomously, learn from data, adapt to new situations, and improve performance over time without explicit programming.
Techniques and methods
Big Data involves technologies and techniques for data collection, storage, processing, and analysis, such as distributed computing, parallel processing, data mining, machine learning, and advanced analytics.
AI encompasses various subfields and techniques, including machine learning, deep learning, natural language processing (NLP), computer vision, expert systems, and robotics, among others.
Relationship
Here’s where AI and big data intersect. AI often relies on big data to train its models and algorithms. Large datasets are essential for training machine learning and deep learning models to recognize patterns and make accurate predictions.
Big data analytics can also be enhanced by incorporating AI techniques for more advanced analysis, such as predictive analytics, sentiment analysis, anomaly detection, and personalized recommendations.
Big Data Use Cases
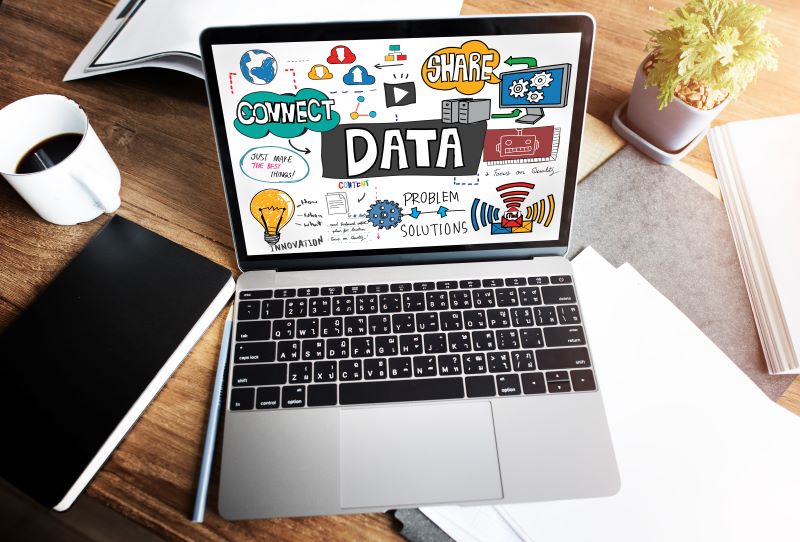
Big data and AI both have a wide range of applications across many industries. Here are some common use cases for big data:
Business and finance
Big data analytics can analyze vast amounts of financial transactions to identify patterns that might indicate fraud. This helps banks and credit card companies prevent fraudulent activity.
Companies can use big data to understand their customers better, too, including their preferences, buying habits, and online behavior. This allows for targeted marketing campaigns, product recommendations, and improved customer service.
Big data also helps financial institutions assess and manage risks associated with lending, investing, and other financial activities.
Healthcare
By analyzing a patient’s medical history, genetic data, and lifestyle factors, doctors can design personalized treatment plans that are more effective.
Big data can also be used to identify patients at high risk for certain diseases, allowing for early intervention and prevention.
Researchers can analyze vast datasets to identify potential new drugs and therapies as well.
Retail and e-commerce
Big data helps retailers predict demand for products and optimize their inventory levels. This reduces the risk of stockouts and overstocking.
By analyzing past sales data and customer trends, retailers can also forecast future demand and plan their inventory and marketing strategies accordingly.
e-commerce platforms use big data to recommend products to customers based on their past purchases and browsing behavior, too.
AI Use Cases
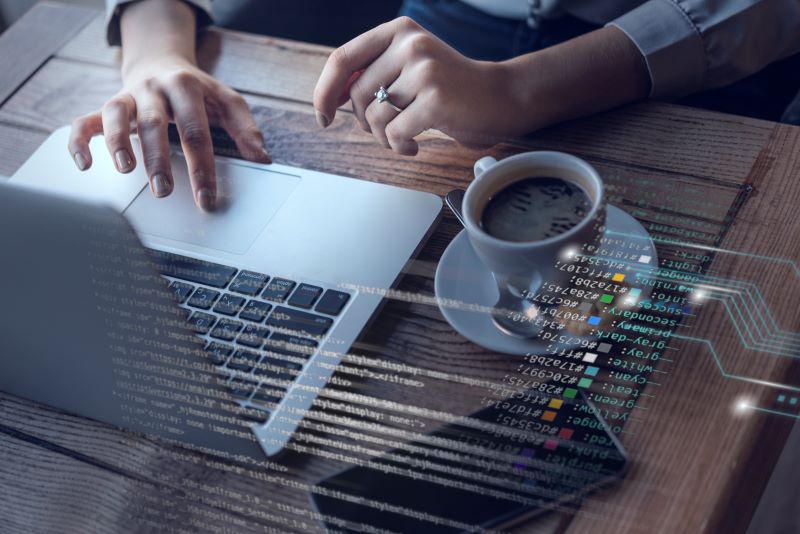
Artificial intelligence is having a transformative impact on a wide range of industries. Here are some of the most prominent examples:
Healthcare
AI is being used in healthcare to diagnose diseases, develop new drugs, and personalize treatment plans. For instance, AI-powered systems can analyze medical scans to detect abnormalities and suggest treatment options.
Finance
AI is also used for fraud detection, algorithmic trading, and risk management in the financial sector. It can analyze incredible amounts of financial data to identify suspicious transactions and make informed investment decisions.
Manufacturing
AI is revolutionizing manufacturing by optimizing production lines, predicting equipment failures, and improving quality control. Robots powered by AI can perform complex tasks with greater precision and efficiency.
Retail
AI is used in retail to personalize recommendations, optimize inventory management, and improve customer service. For example, AI-powered chatbots can answer customer questions and provide product recommendations.
Transportation
AI is being used to develop self-driving cars and improve traffic management. Self-driving cars rely on AI for perception, navigation, and decision-making. AI algorithms process sensor data to navigate roads, avoid obstacles, and ensure passenger safety.
Customer service
AI-powered chatbots are used to provide customer service by answering questions, resolving issues, and directing customers to the appropriate resources.
Media and entertainment
AI is used to personalize content recommendations, generate creative content, and power virtual reality experiences. For example, AI-powered algorithms can recommend movies or TV shows that you might enjoy based on your past viewing history.
How Can AI Be Used in Web Scraping?
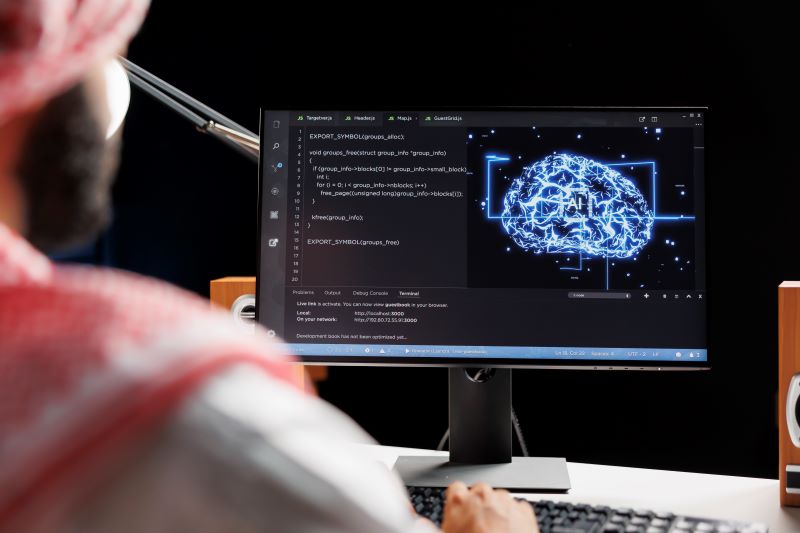
As you can see, big data and AI are bringing major advancements and making impressive contributions to a wide range of industries and sectors. The world of web scraping is no exception.
Web scraping is the process of using software to extract data from websites and organize it in a way that’s more usable.
Here’s how AI is transforming web scraping:
- Adaptability: Unlike traditional scripts that rely on fixed website structures, AI-powered scrapers can adapt to changing website layouts. AI uses techniques like computer vision to understand the visual elements on a webpage and extract data regardless of minor structural changes.
- Anti-scraping measures: Websites often employ anti-scraping measures like CAPTCHAs and IP blocking to deter scraping bots. AI scrapers can mimic human behavior, including browsing patterns and mouse movements, to bypass these measures. Additionally, AI can leverage proxies to switch IP addresses and appear like multiple users.
- Data accuracy and efficiency: AI can significantly improve the accuracy and efficiency of web scraping. AI algorithms can identify the relevant data points on a webpage with high precision, reducing errors and the need for manual intervention.
- Complex data handling: AI can handle complex data formats beyond simple text. AI can process and extract data from images, videos, and other multimedia content found on web pages.
AI can also be implemented in a variety of web scraping tools. For example, an AI program manager might use machine learning algorithms to learn from past scraping experiences and improve their ability to identify and extract data patterns over time.
Natural Language Processing also allows AI to understand the context and meaning of text data on web pages. This is useful for tasks like sentiment analysis or extracting specific details from product descriptions.
Deep learning algorithms, inspired by the structure of the human brain, can also be particularly effective in tasks like image recognition, enabling AI to extract data from images and videos on webpages.
Big Data’s Role in Web Scraping
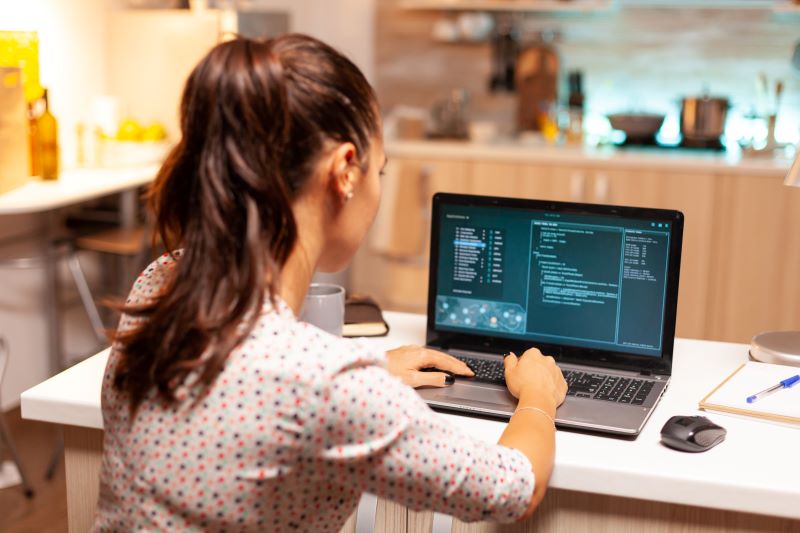
What about big data?
Big data acts like a powerful behind-the-scenes player that supercharges web scraping operations in several ways, including the following:
Finding hidden gems
Big data analytics shines in helping you identify valuable data sources. By sifting through mountains of information online, it can pinpoint websites likely to hold the data you need. Imagine analyzing search trends or social media conversations to find websites relevant to your scraping goals.
Prioritizing efforts
Not all data is created equal. Big data helps you prioritize which websites to scrape first. It considers factors like data freshness, update frequency, and potential scraping difficulty. This way, you can optimize your strategy and target the most valuable sources first.
Organizing massive amounts of data
Big data technologies are essential for storing and managing the massive amount of data you collect through web scraping. Distributed file systems and cloud storage solutions can handle this immense volume efficiently.
Filtering and cleaning data
Big data processing tools come in handy after the scraping is done. For example, a database management company will often use them to filter and clean data, remove irrelevant information, identify inconsistencies, and get it ready for further analysis. Techniques like anomaly detection can also be used to flag suspicious data points.
Identifying hidden patterns and trends
Once you have a large collection of scraped data, big data analytics tools can work their magic. They can uncover hidden patterns and trends that might be invisible in smaller datasets. This can provide valuable insights that can inform business decisions or research endeavors.
For example, a company researching travel trends can leverage big data to identify popular destinations based on website traffic data. Then, they could scrape travel websites for hotel prices, flight options, and user reviews in those specific locations. By analyzing this scraped data, they can discover trends like seasonal pricing fluctuations or user preferences for different types of accommodations.
AI and Proxies
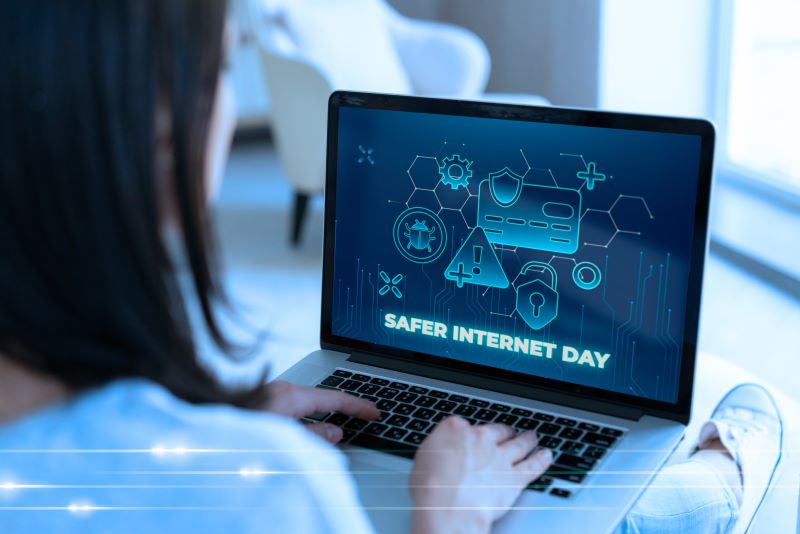
AI and proxies can be a powerful duo for web scraping, but it’s important to understand both the benefits and potential drawbacks. Here’s how AI can leverage proxies for more effective and efficient web scraping:
Bypassing anti-scraping measures
AI can be used to manage a pool of proxies and rotate them intelligently. This helps avoid getting blocked by websites that employ IP blacklisting to identify and stop scraping bots.
AI can also analyze real user browsing patterns and use this knowledge to make scraping requests appear more human-like. This includes things like varying time delays between requests and simulating mouse movements.
Extracting complex data
Websites can change their layout dynamically. AI can use computer vision to understand the visual elements on a webpage and adjust the scraping process on the fly to extract the target data regardless of minor structural changes.
Data filtering and quality enhancement
AI can process the scraped data and identify inconsistencies or anomalies that might indicate irrelevant or inaccurate information. This helps improve the overall quality of the scraped dataset.
Big Data and Proxies
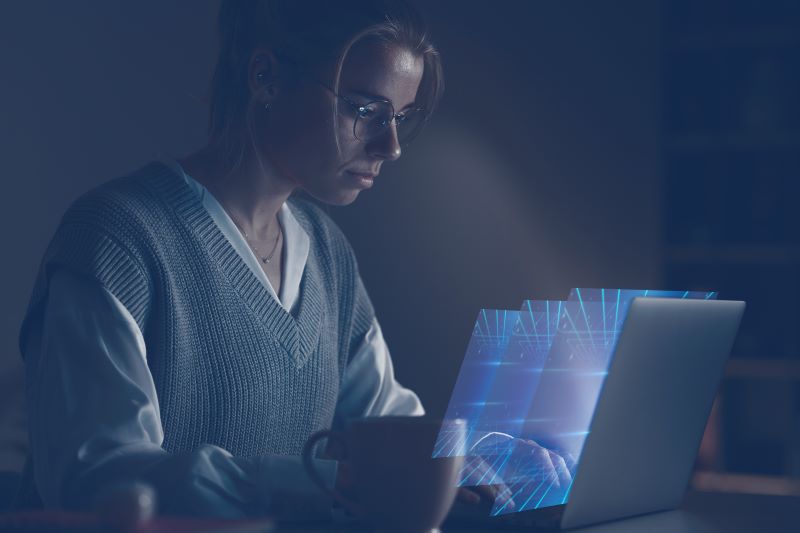
The relationship between big data and proxies primarily exists in the context of data collection, analysis, and interpretation.
Proxies are often used in situations where direct measurement of a particular variable is difficult, costly, or impractical. In such cases, proxies serve as substitutes or indirect indicators for the variable of interest.
Here’s some more insight into how big data and proxies relate:
Data collection
Big data often involves collecting large amounts of data from various sources. Proxies can be used to supplement or represent certain data points that are challenging to obtain directly. For example, in financial markets, stock prices might serve as proxies for market sentiment or economic health.
Data enrichment
Proxies can enrich big data sets by providing additional contextual information. For instance, demographic information such as age, gender, and location can act as proxies for consumer behavior patterns, helping to segment and analyze customer data more effectively.
Analysis and prediction
Big data analytics often involves identifying patterns, trends, and correlations within large datasets. Proxies can be used to uncover hidden relationships or predict outcomes when direct measurements are unavailable or incomplete. For instance, search engine queries might serve as proxies for public interest or sentiment on specific topics.
Risk management
In various domains, such as finance, insurance, and cybersecurity, proxies are used to assess and manage risks. For example, credit scores serve as proxies for creditworthiness in lending decisions, while network traffic patterns can act as proxies for cyber threats.
Privacy preservation
In some cases, proxies are employed to protect individual privacy while still enabling data analysis. Aggregated or anonymized data can serve as proxies for individual-level data, allowing organizations to derive insights without compromising personal information.
Modeling complex systems
Proxies are often employed in modeling complex systems where direct measurement of all variables is impractical. In climate science, for example, temperature measurements from weather stations serve as proxies for broader climate trends.
Final Thoughts
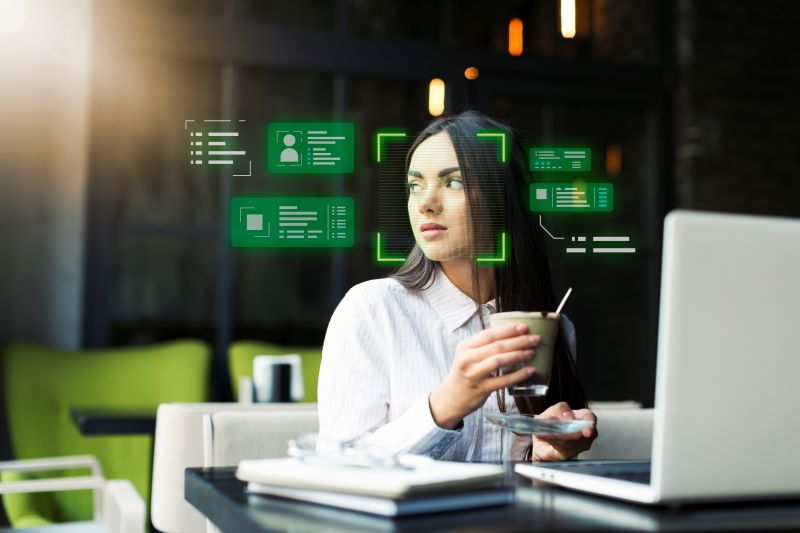
You don’t have to pick a side between big data vs. AI. Both big data and AI can work together — along with other tools like proxies — to produce better outcomes, especially when carrying out tasks like web scraping.
Don’t end your learning journey at the differences between big data vs. AI. Check out some of Rayobyte’s other blog posts for more insight. This one on web scraping for beginners is a great option for continuing to learn about web scraping best practices.
The information contained within this article, including information posted by official staff, guest-submitted material, message board postings, or other third-party material is presented solely for the purposes of education and furtherance of the knowledge of the reader. All trademarks used in this publication are hereby acknowledged as the property of their respective owners.